Learners will implement and apply predictive, classification, clustering, and information retrieval machine learning algorithms to real datasets throughout each course in the specialization. They will walk away with applied machine learning and Python programming experience.
Thursday, 30 March 2017
Tuesday, 28 March 2017
Friday, 24 March 2017
Friday, 17 March 2017
Internshala VTC Young Achiever Scholarship for your students
Dayananda Sagar College of Engineering
Internshala VTC Young Achiever Scholarship for your students
Internshala launched the 3rd edition of Internshala VTC Young Achiever Scholarship.
This initiative by Internshala VTC (Virtual Training Center) helps bright students who have the desire to learn and excel in their career but may not have adequate resources.
Under this scholarship program, Internshala is giving free training programs worth Rs 2 lacs to 50 students.
Who can participate?
- who are pursuing graduation or post-graduation
- whose family income is less than 2 lacs per annum
Students need to register here - internshala.com/i/2239 to participate (registrations have already begun). The last date to register is 26th March.
Thursday, 16 March 2017
Origami - Unfolding the Folds
Dayananda Sagar College of Engineering
Origami - Unfolding the Folds
The Department of Mathematics is conducting a workshop on
“Origami – Unfolding the Folds” on 20th March,2017 in CD Sagar
auditorium between 2pm-4.30pm.
Most
people who know Origami think of it as the art of paper folding. Though it
began centuries ago, Origami became better known to the world in the 20th
century when it evolved in to a modern art form. In 21st century,
Origami has caught the attention of engineers who are using it to create all
sorts of new structures – from collapsible packaging to airbags. Origami has
even found its way in to space.
The Objective of the Workshop is to equip the students with
basic principles of folds in an Origami with hands on session of making
Geometric models.
The Student can also portray Origami Models for which they
will be rewarded “Best Origami model”
under the Category. Good models can be considered for AAT 2.
- Engineering Model
- Geometric Model
All the students are cordially invited to attend the
workshop on “Origami – Unfolding the Folds”
Students who have registered will be given attendance for the duration of the workshop. Only first 150 entries will be entertained.
Students who have registered will be given attendance for the duration of the workshop. Only first 150 entries will be entertained.
You can register for the workshop below.
- Last date of Registration: 19/3/2017
Wednesday, 15 March 2017
Saturday, 11 March 2017
One Day Seminar on Ethical Hacking
Dayananda Sagar College of Engineering
One Day Seminar on Ethical Hacking
Ever heard of Hacker's, The Ghost in the wires, people who know the ins and outs of a secured system?
Ever wanted to become one?
Here's your chance.
We are organizing a one day seminar on Ethical Hacking and Web Application Security on 25th of March at 2:00 pm.

It will be focused on Ethical Hacking, Bug bounty programs and the nit-bits of Web application Security.
Aditya Dixit will be presenting the seminar, who ranks among top 500 bug bounty hunters globally on Bugcrowd and is also in the core security team of Cobalt.
Various methods, tools, and vulnerabilities will be discussed including different kinds of bugs which exist in modern day applications.
Registrations are closed. Further information will be e-mailed to the registered users.
For more information contact Aditya ~ 8095117619
Wednesday, 8 March 2017
Machine Learning - University of Washington | Coursera
This Specialization from leading researchers at the University of Washington introduces you to the exciting, high-demand field of Machine Learning. Through a series of practical case studies, you will gain applied experience in major areas of Machine Learning including Prediction, Classification, Clustering, and Information Retrieval. You will learn to analyze large and complex datasets, create systems that adapt and improve over time, and build intelligent applications that can make predictions from data.
Created by:
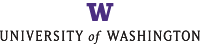
Industry Partners:
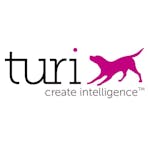
4 courses
Follow the suggested order or choose your own.
Projects
Designed to help you practice and apply the skills you learn.
Certificates
Highlight your new skills on your resume or LinkedIn.
Projects Overview
Courses
- Intermediate Specialization.
- Some related experience required.
COURSE 1
Machine Learning Foundations: A Case Study Approach
Current session: Mar 6 — Apr 24.- Commitment
- 6 weeks of study, 5-8 hours/week
- Subtitles
- English, Korean, Chinese (Simplified)
About the Course
Do you have data and wonder what it can tell you? Do you need a deeper understanding of the core ways in which machine learning can improve your business? Do you want to be able to converse with specialists about anything from regression and classification to deep learning and recommender systems? In this course, you will get hands-on experience with machine learning from a series of practical case-studies. At the end of the first course you will have studied how to predict house prices based on house-level features, analyze sentiment from user reviews, retrieve documents of interest, recommend products, and search for images. Through hands-on practice with these use cases, you will be able to apply machine learning methods in a wide range of domains. This first course treats the machine learning method as a black box. Using this abstraction, you will focus on understanding tasks of interest, matching these tasks to machine learning tools, and assessing the quality of the output. In subsequent courses, you will delve into the components of this black box by examining models and algorithms. Together, these pieces form the machine learning pipeline, which you will use in developing intelligent applications. Learning Outcomes: By the end of this course, you will be able to: -Identify potential applications of machine learning in practice. -Describe the core differences in analyses enabled by regression, classification, and clustering. -Select the appropriate machine learning task for a potential application. -Apply regression, classification, clustering, retrieval, recommender systems, and deep learning. -Represent your data as features to serve as input to machine learning models. -Assess the model quality in terms of relevant error metrics for each task. -Utilize a dataset to fit a model to analyze new data. -Build an end-to-end application that uses machine learning at its core. -Implement these techniques in Python.Show or hide details about course Machine Learning Foundations: A Case Study ApproachCOURSE 2
Machine Learning: Regression
Current session: Mar 6 — Apr 24.- Commitment
- 6 weeks of study, 5-8 hours/week
- Subtitles
- English
About the Course
Case Study - Predicting Housing Prices In our first case study, predicting house prices, you will create models that predict a continuous value (price) from input features (square footage, number of bedrooms and bathrooms,...). This is just one of the many places where regression can be applied. Other applications range from predicting health outcomes in medicine, stock prices in finance, and power usage in high-performance computing, to analyzing which regulators are important for gene expression. In this course, you will explore regularized linear regression models for the task of prediction and feature selection. You will be able to handle very large sets of features and select between models of various complexity. You will also analyze the impact of aspects of your data -- such as outliers -- on your selected models and predictions. To fit these models, you will implement optimization algorithms that scale to large datasets. Learning Outcomes: By the end of this course, you will be able to: -Describe the input and output of a regression model. -Compare and contrast bias and variance when modeling data. -Estimate model parameters using optimization algorithms. -Tune parameters with cross validation. -Analyze the performance of the model. -Describe the notion of sparsity and how LASSO leads to sparse solutions. -Deploy methods to select between models. -Exploit the model to form predictions. -Build a regression model to predict prices using a housing dataset. -Implement these techniques in Python.Show or hide details about course Machine Learning: RegressionCOURSE 3
Machine Learning: Classification
Current session: Mar 6 — May 1.- Commitment
- 7 weeks of study, 5-8 hours/week
- Subtitles
- English
About the Course
Case Studies: Analyzing Sentiment & Loan Default Prediction In our case study on analyzing sentiment, you will create models that predict a class (positive/negative sentiment) from input features (text of the reviews, user profile information,...). In our second case study for this course, loan default prediction, you will tackle financial data, and predict when a loan is likely to be risky or safe for the bank. These tasks are an examples of classification, one of the most widely used areas of machine learning, with a broad array of applications, including ad targeting, spam detection, medical diagnosis and image classification. In this course, you will create classifiers that provide state-of-the-art performance on a variety of tasks. You will become familiar with the most successful techniques, which are most widely used in practice, including logistic regression, decision trees and boosting. In addition, you will be able to design and implement the underlying algorithms that can learn these models at scale, using stochastic gradient ascent. You will implement these technique on real-world, large-scale machine learning tasks. You will also address significant tasks you will face in real-world applications of ML, including handling missing data and measuring precision and recall to evaluate a classifier. This course is hands-on, action-packed, and full of visualizations and illustrations of how these techniques will behave on real data. We've also included optional content in every module, covering advanced topics for those who want to go even deeper! Learning Objectives: By the end of this course, you will be able to: -Describe the input and output of a classification model. -Tackle both binary and multiclass classification problems. -Implement a logistic regression model for large-scale classification. -Create a non-linear model using decision trees. -Improve the performance of any model using boosting. -Scale your methods with stochastic gradient ascent. -Describe the underlying decision boundaries. -Build a classification model to predict sentiment in a product review dataset. -Analyze financial data to predict loan defaults. -Use techniques for handling missing data. -Evaluate your models using precision-recall metrics. -Implement these techniques in Python (or in the language of your choice, though Python is highly recommended).Show or hide details about course Machine Learning: ClassificationCOURSE 4
Machine Learning: Clustering & Retrieval
Current session: Mar 6 — Apr 24.- Commitment
- 6 weeks of study, 5-8 hours/week
- Subtitles
- English
About the Course
Case Studies: Finding Similar Documents A reader is interested in a specific news article and you want to find similar articles to recommend. What is the right notion of similarity? Moreover, what if there are millions of other documents? Each time you want to a retrieve a new document, do you need to search through all other documents? How do you group similar documents together? How do you discover new, emerging topics that the documents cover? In this third case study, finding similar documents, you will examine similarity-based algorithms for retrieval. In this course, you will also examine structured representations for describing the documents in the corpus, including clustering and mixed membership models, such as latent Dirichlet allocation (LDA). You will implement expectation maximization (EM) to learn the document clusterings, and see how to scale the methods using MapReduce. Learning Outcomes: By the end of this course, you will be able to: -Create a document retrieval system using k-nearest neighbors. -Identify various similarity metrics for text data. -Reduce computations in k-nearest neighbor search by using KD-trees. -Produce approximate nearest neighbors using locality sensitive hashing. -Compare and contrast supervised and unsupervised learning tasks. -Cluster documents by topic using k-means. -Describe how to parallelize k-means using MapReduce. -Examine probabilistic clustering approaches using mixtures models. -Fit a mixture of Gaussian model using expectation maximization (EM). -Perform mixed membership modeling using latent Dirichlet allocation (LDA). -Describe the steps of a Gibbs sampler and how to use its output to draw inferences. -Compare and contrast initialization techniques for non-convex optimization objectives. -Implement these techniques in Python.Show or hide details about course Machine Learning: Clustering & Retrieval
Creators
The University of Washington is a national and international leader in the core fields that are driving data science: computer science, statistics, human-centered design, and applied math.Founded in 1861, the University of Washington is one of the oldest state-supported institutions of higher education on the West Coast and is one of the preeminent research universities in the world.
Emily Fox
Amazon Professor of Machine Learning
Carlos Guestrin
Amazon Professor of Machine Learning
For more details on any of the courses, visit on www.coursera.org
Subscribe to:
Posts (Atom)